%
PLACEMENT ASSISTANCE
Courses
Students
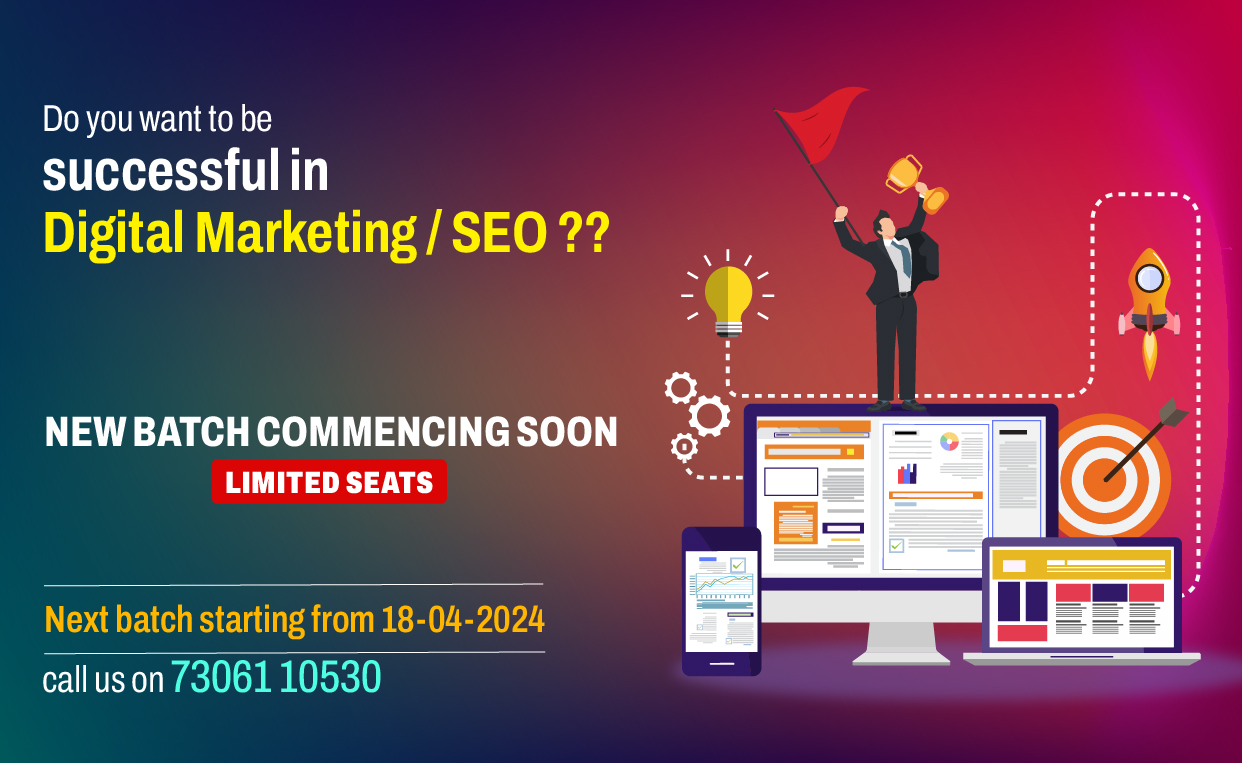
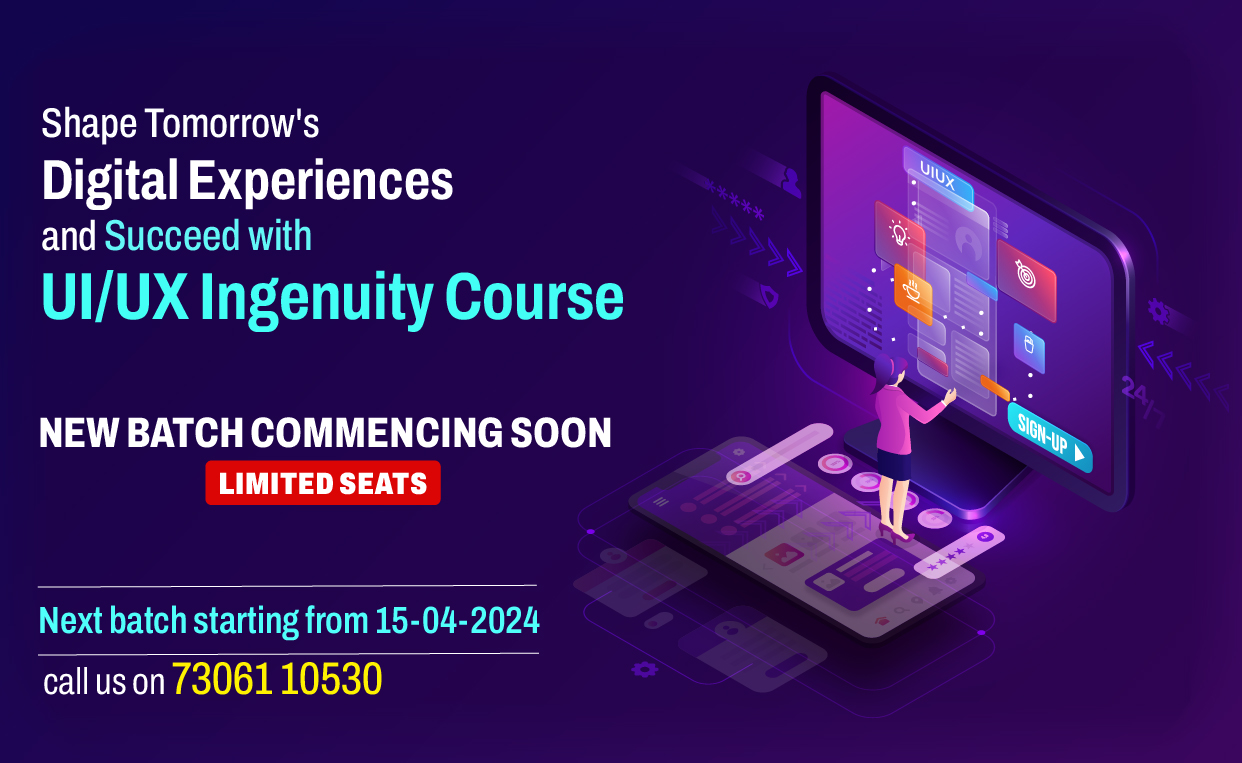
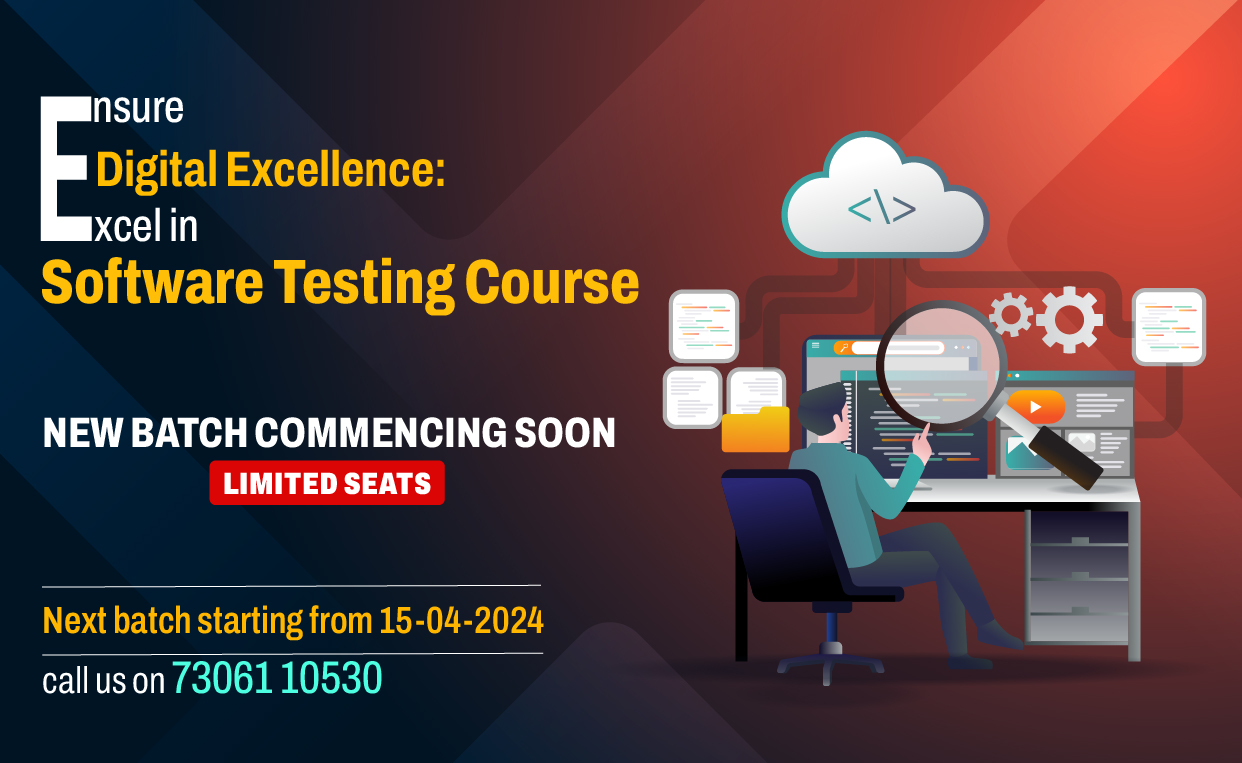
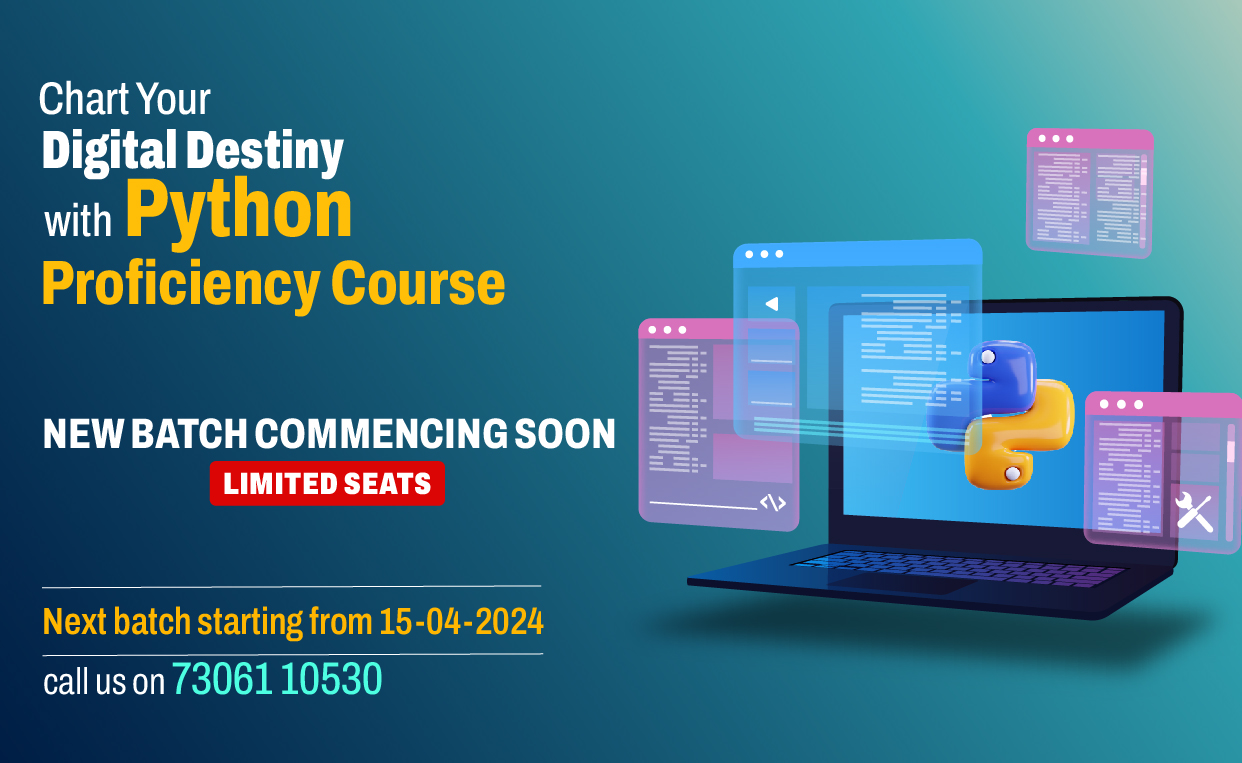
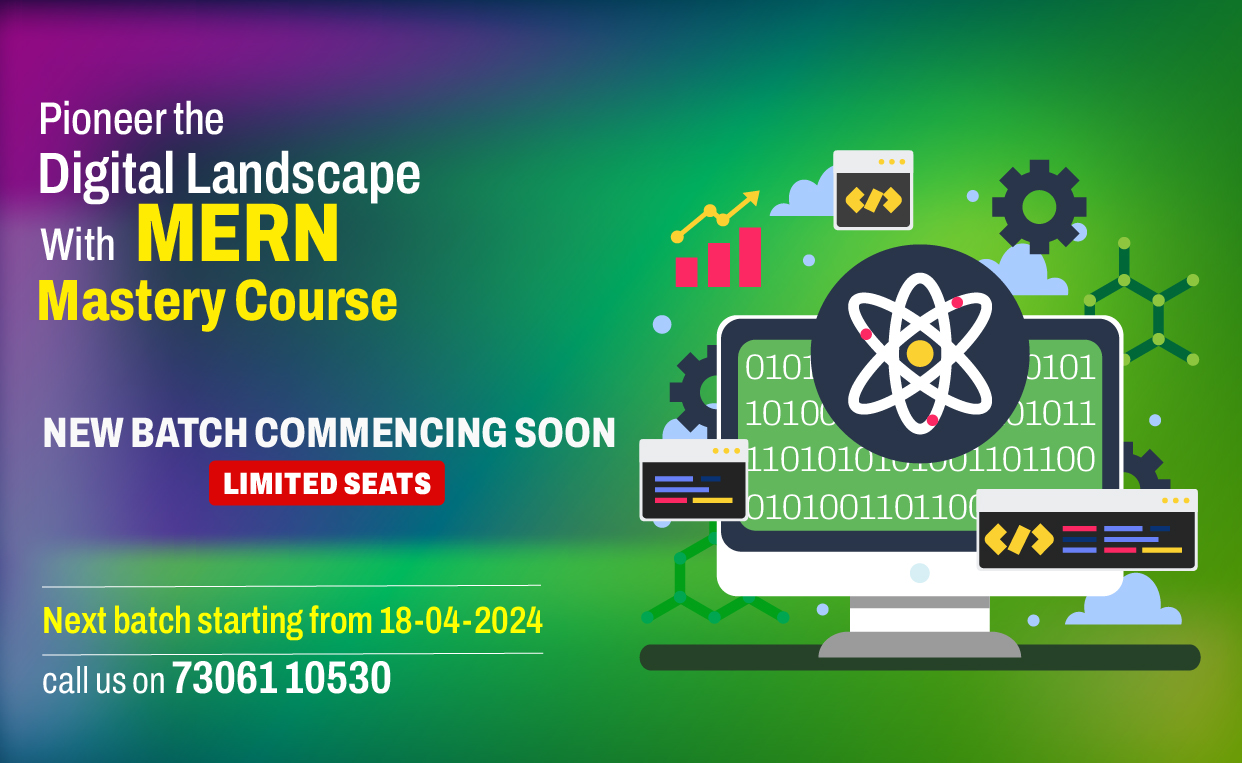
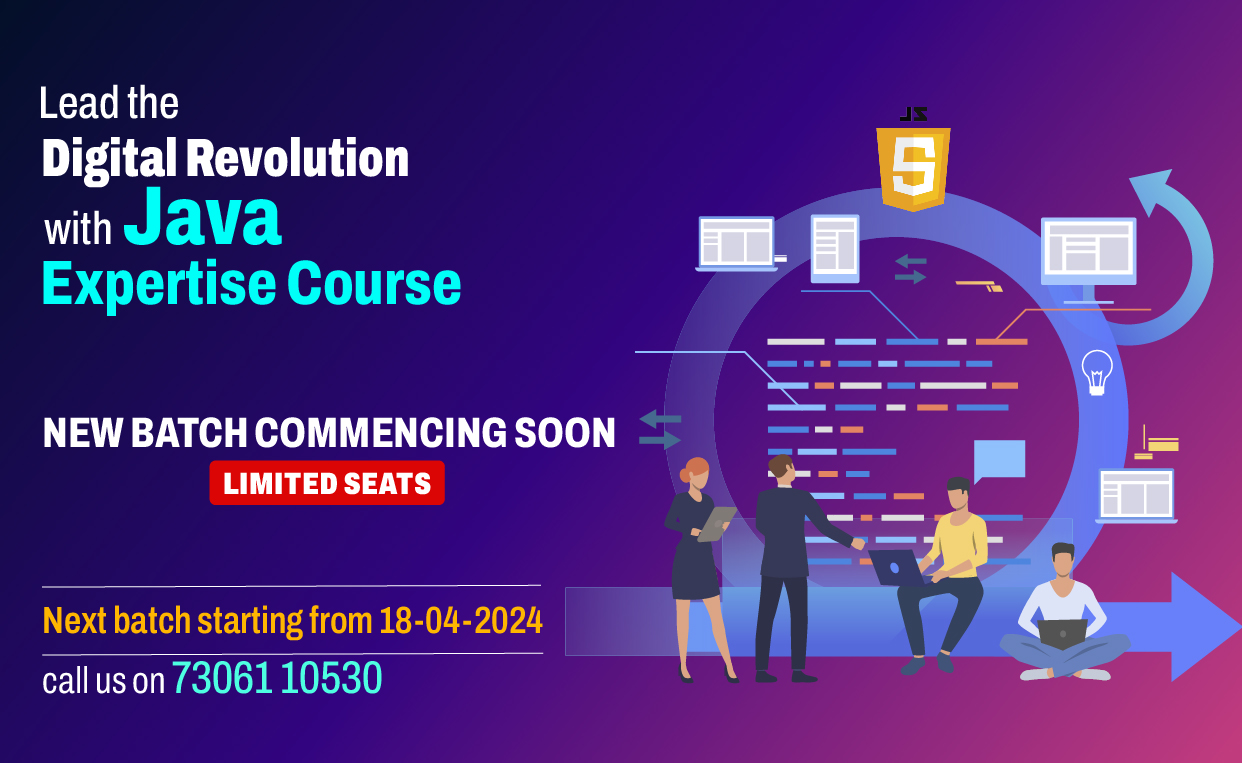
Not sure where to begin?
Our purpose is to extend a helping hand. At our training center, we offer an array of courses and programs aimed at helping you uncover your passions, enhance your skills, and accomplish your aspirations. Whether you’re interested in exploring internship opportunities, student training and corporate training, we have a diverse selection of offerings tailored to meet your needs.
Internship Opportunities
Meaningful, practical work aligned with a student’s field of study or career interest.
Student Training
Training program provides students of all backgrounds the chance to achieve industry-recognized credentials.
Corporate Training
Boost business by empowering employees through professional development and skill enhancement.
Enhance your skills by joining us and get in touch with us today for further information!
Enquire Today!
Uniqueness
What makes Us Special
SLIM Model
SLIM promotes active learner involvement and understanding of learned information, enhancing optimal learning by emphasizing its significance and practical application.
Expert Trainers
Highly experienced trainers along with industry experts to offer practical knowledge and invaluable real-world insights for effective skill development.
Back Up Support
Techmindz, supported by NDimensionZ, a 16-year-old technology company located in Infopark Kochi, is a prominent learning and mentoring platform.
Campus Location
As a global technology company, we have established the first corporate training platform in the central area of Infopark Campus, Kochi.
Success Stories
What our alumni say
Leap to Success
Courses Offered
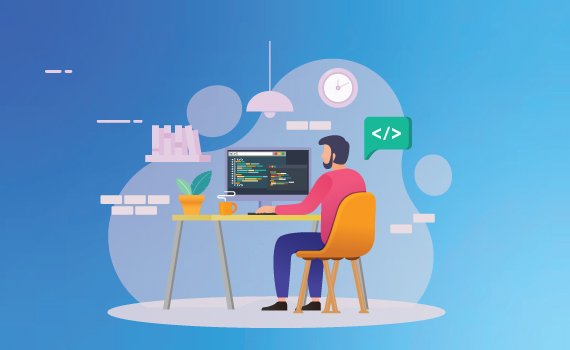
Java
With its versatility and capacity to facilitate a broad range of applications, acquiring knowledge in Java expands your coding possibilities.
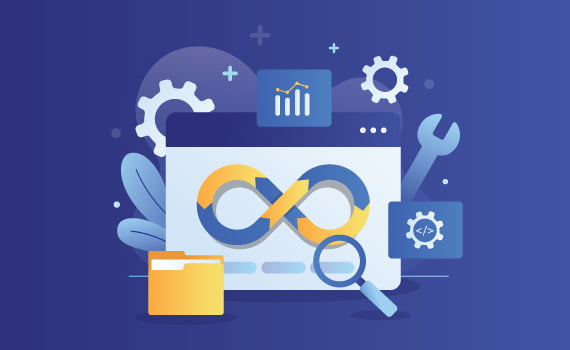
DevOps
DevOps training enhances mastery in continuous development, software development operations, and ongoing integration and delivery of software.
16+
Years Experience
Result Oriented
Corporate Trainings
Techmindz presents a unique training platform that offers professional guidance, preparing individuals for employment opportunities in external companies. Situated at the heart of Infopark Campus, Techmindz stands as the first corporate training platform within an IT firm, providing participants with access to job prospects, internships, and comprehensive technology education within a corporate setting. Our esteemed training panel comprises experienced professionals with a minimum of eight years of expertise in the ever-evolving landscape of technological advancements.
Boosts Employee Retention
Promotes the Organization’s persona
Improves Employee Performance
Helps to Motivate the Employees
Increased Efficiency and Effectiveness
Retention of Top Talent
Certified
Affiliations
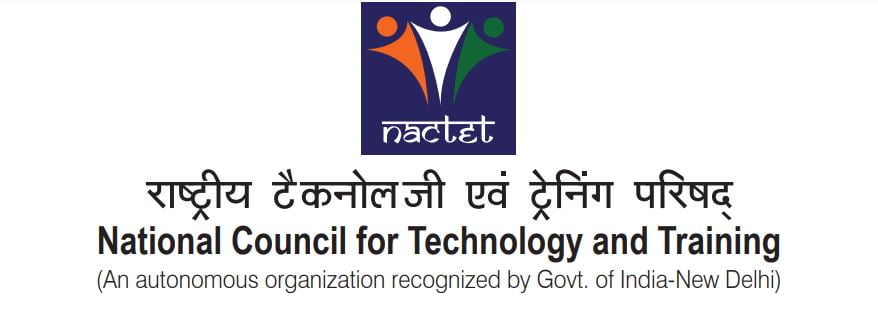
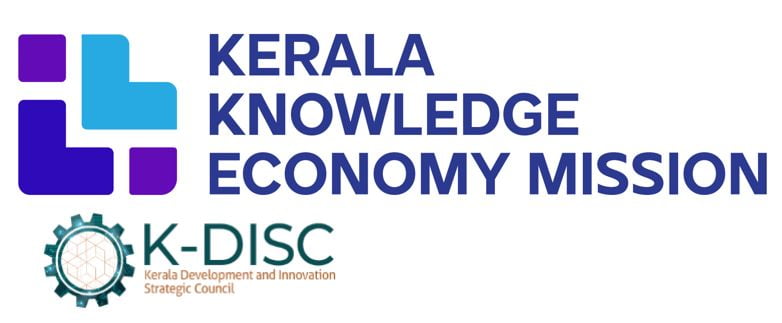
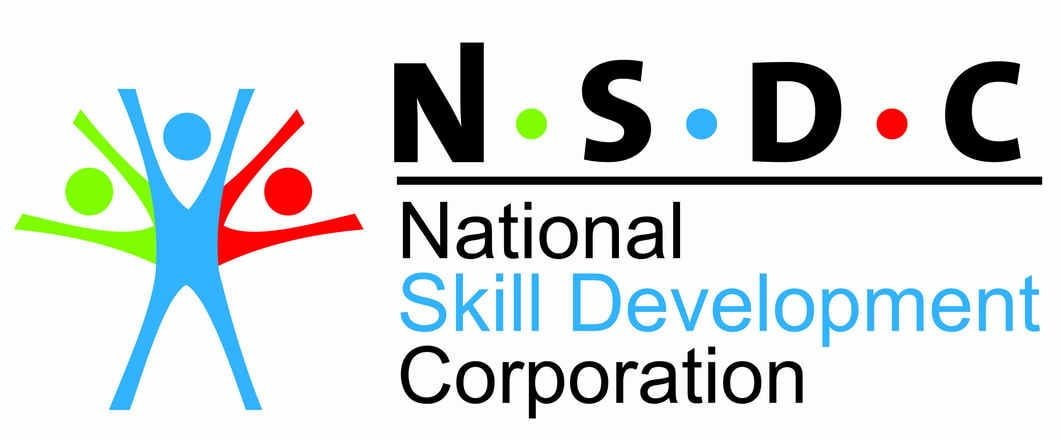
Our Clients
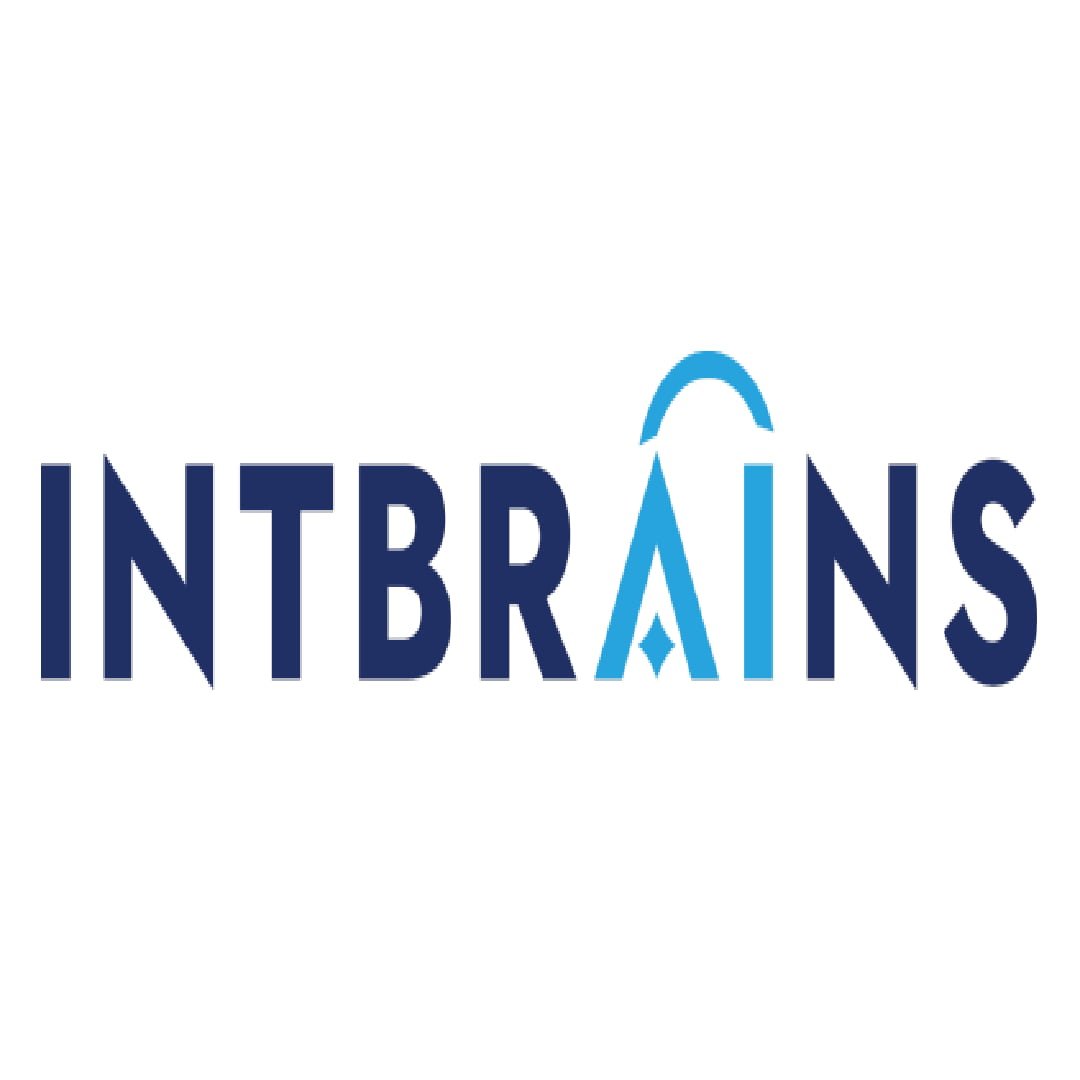
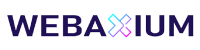
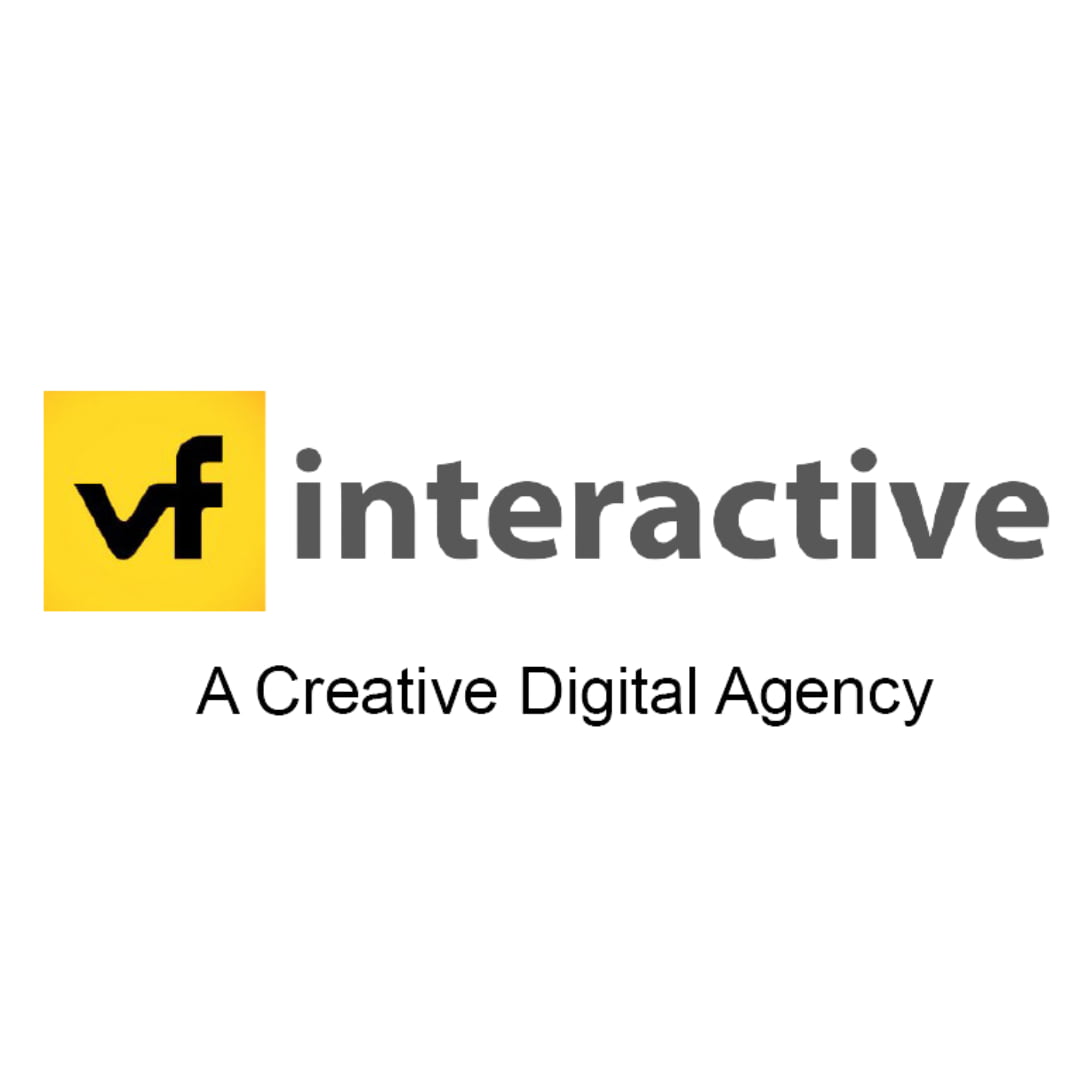
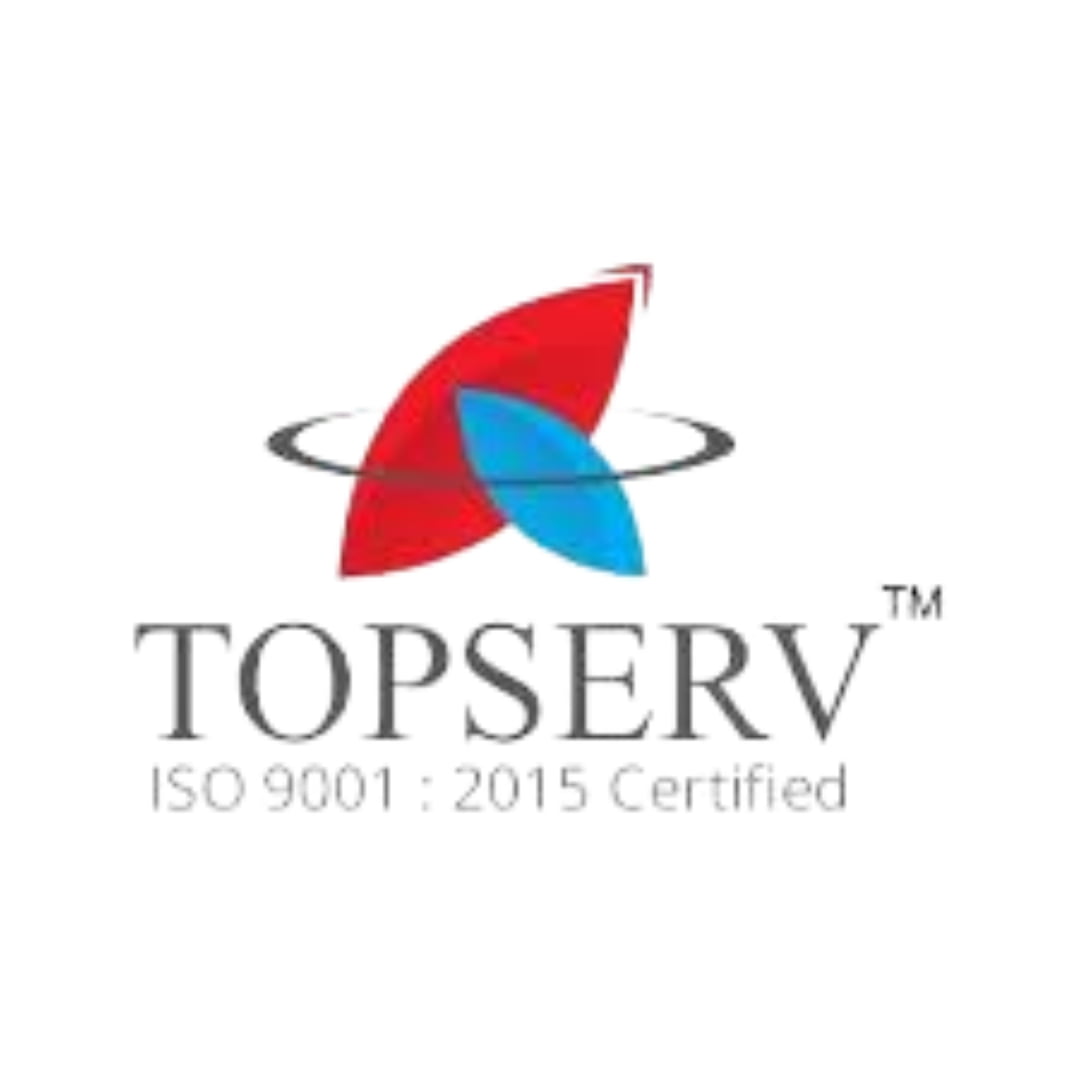
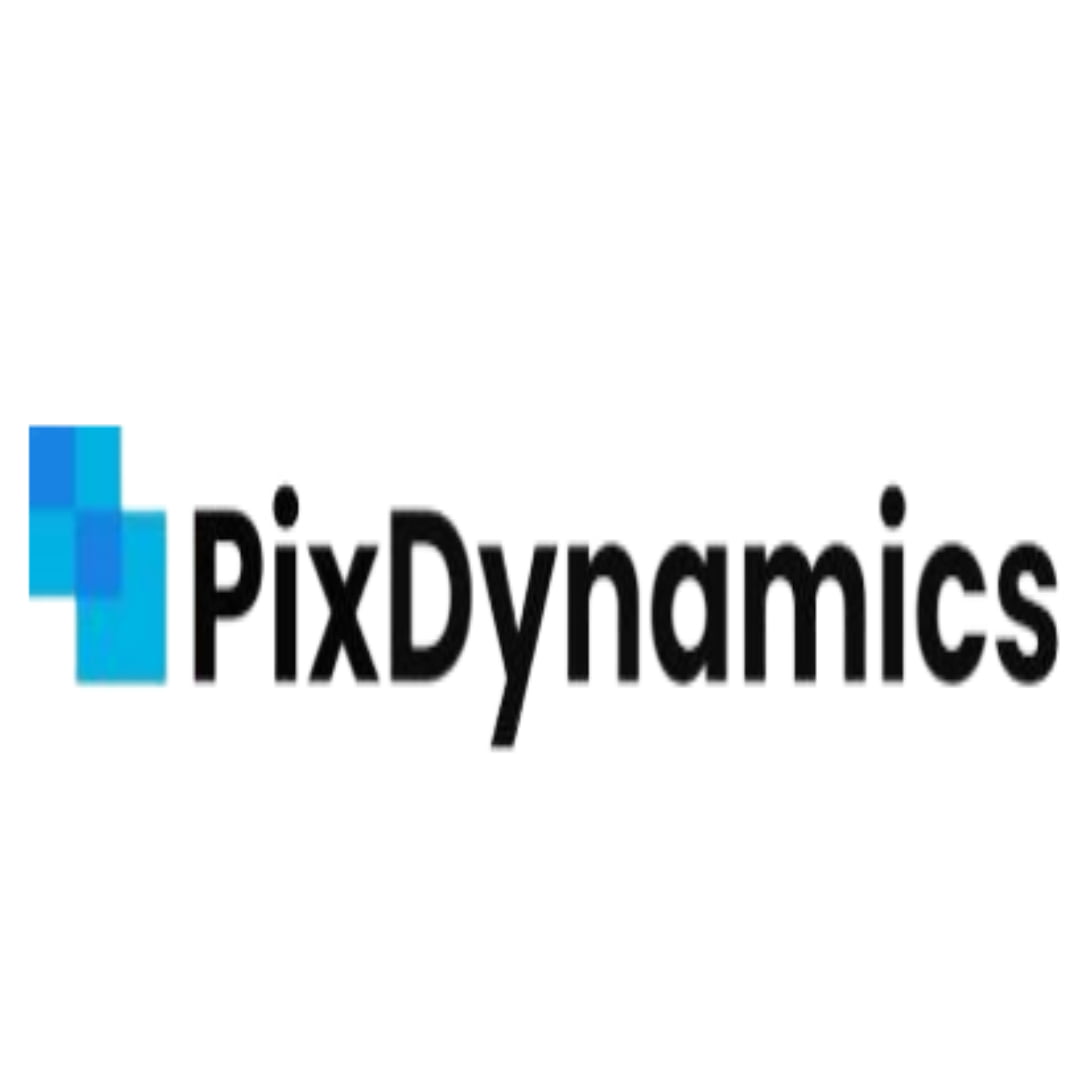
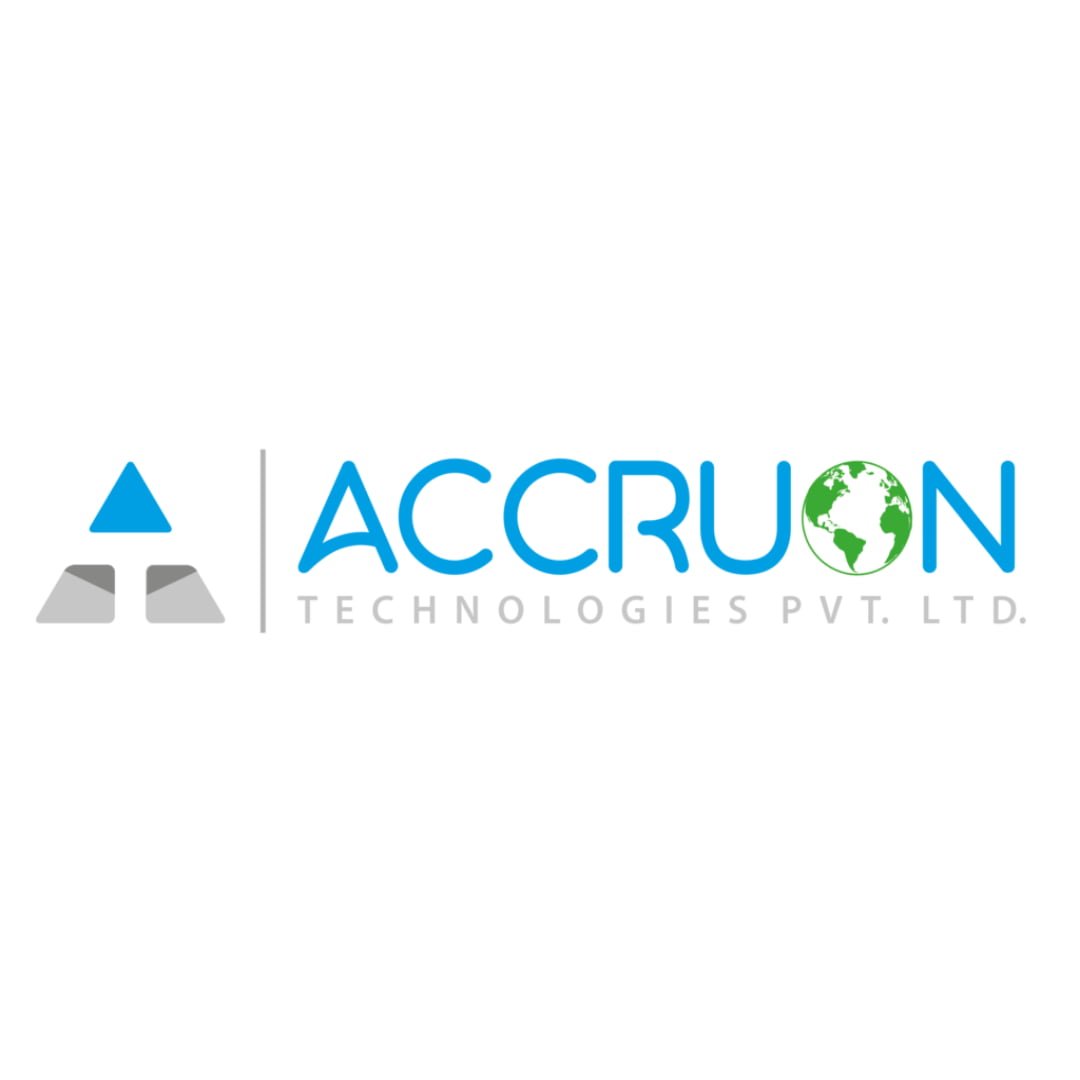
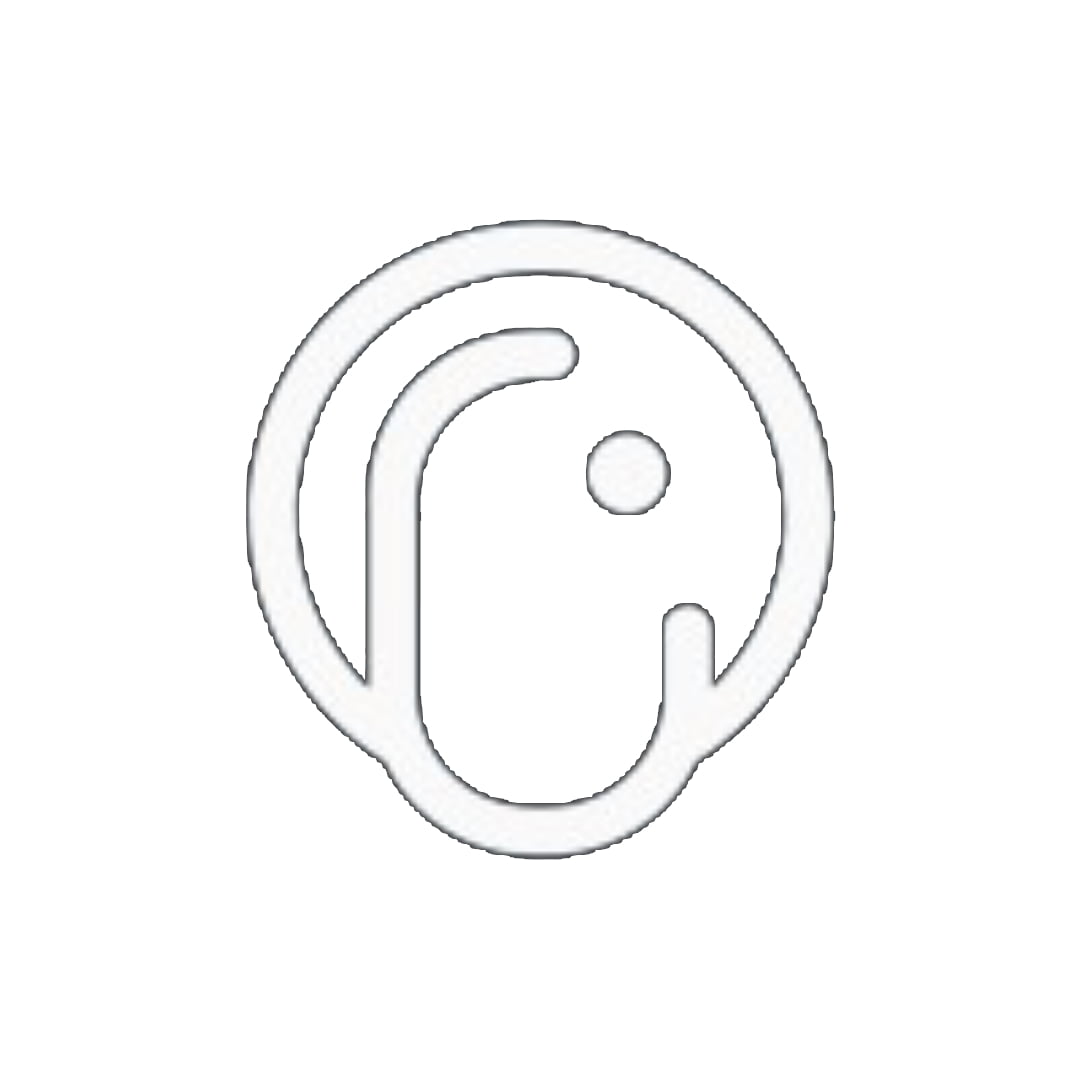
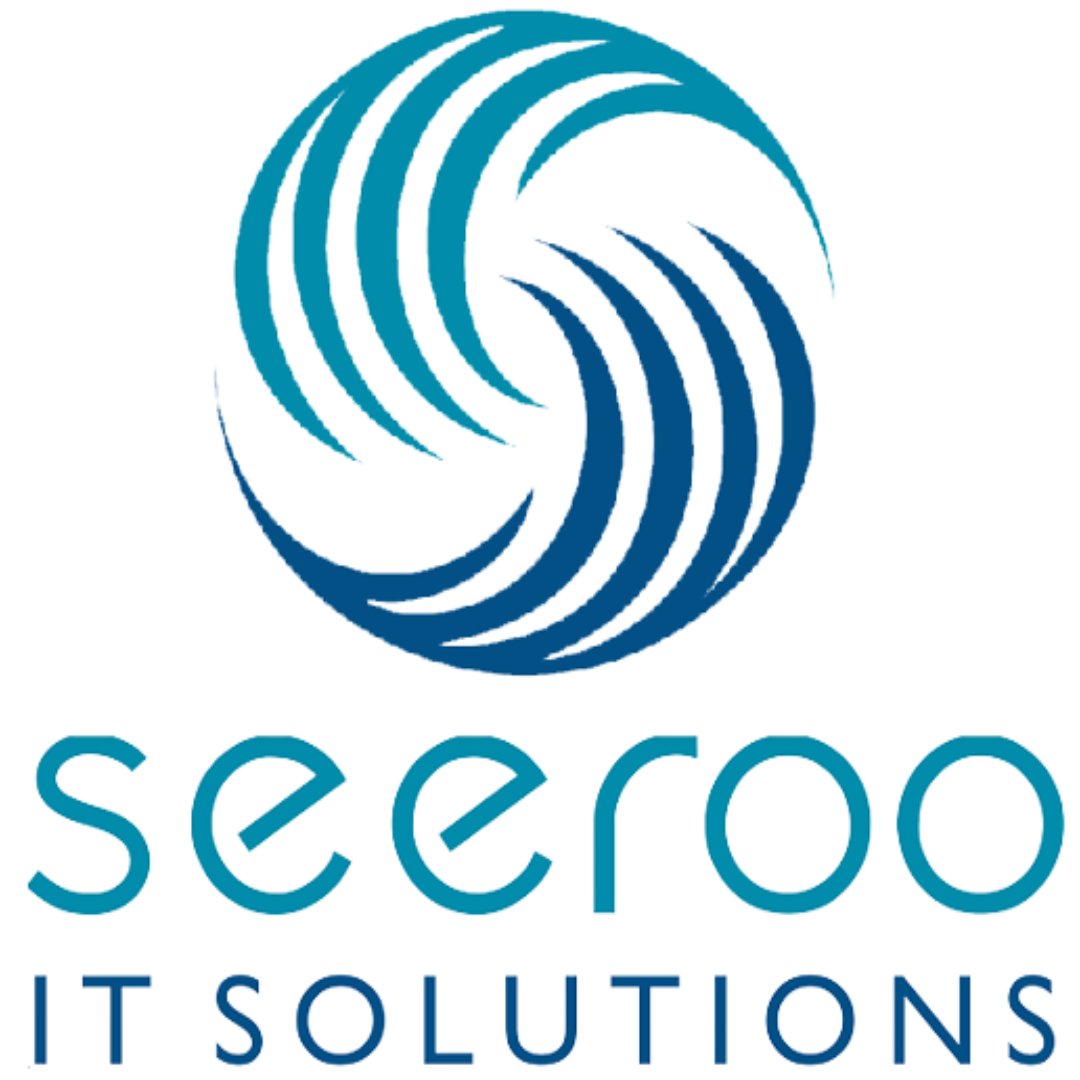
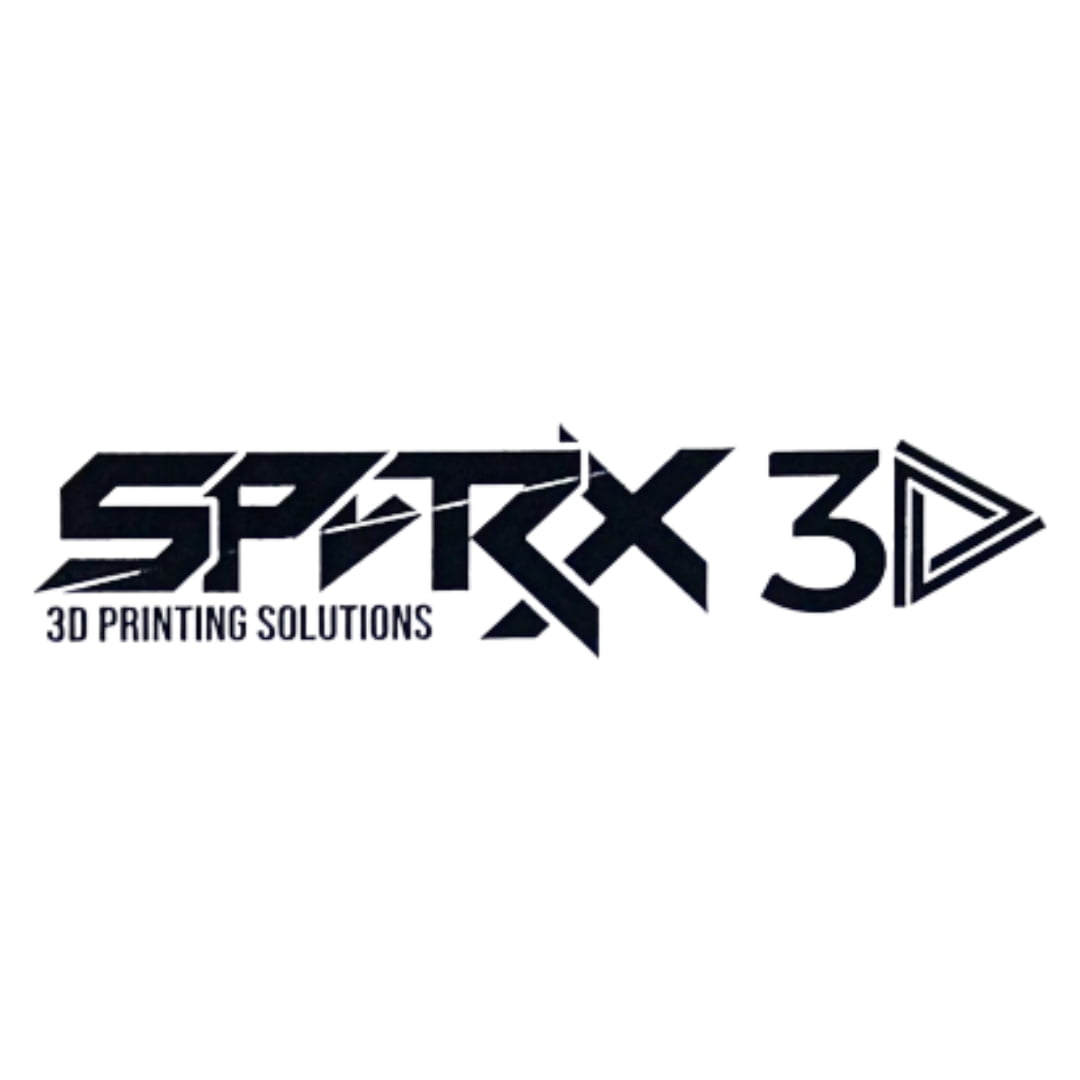
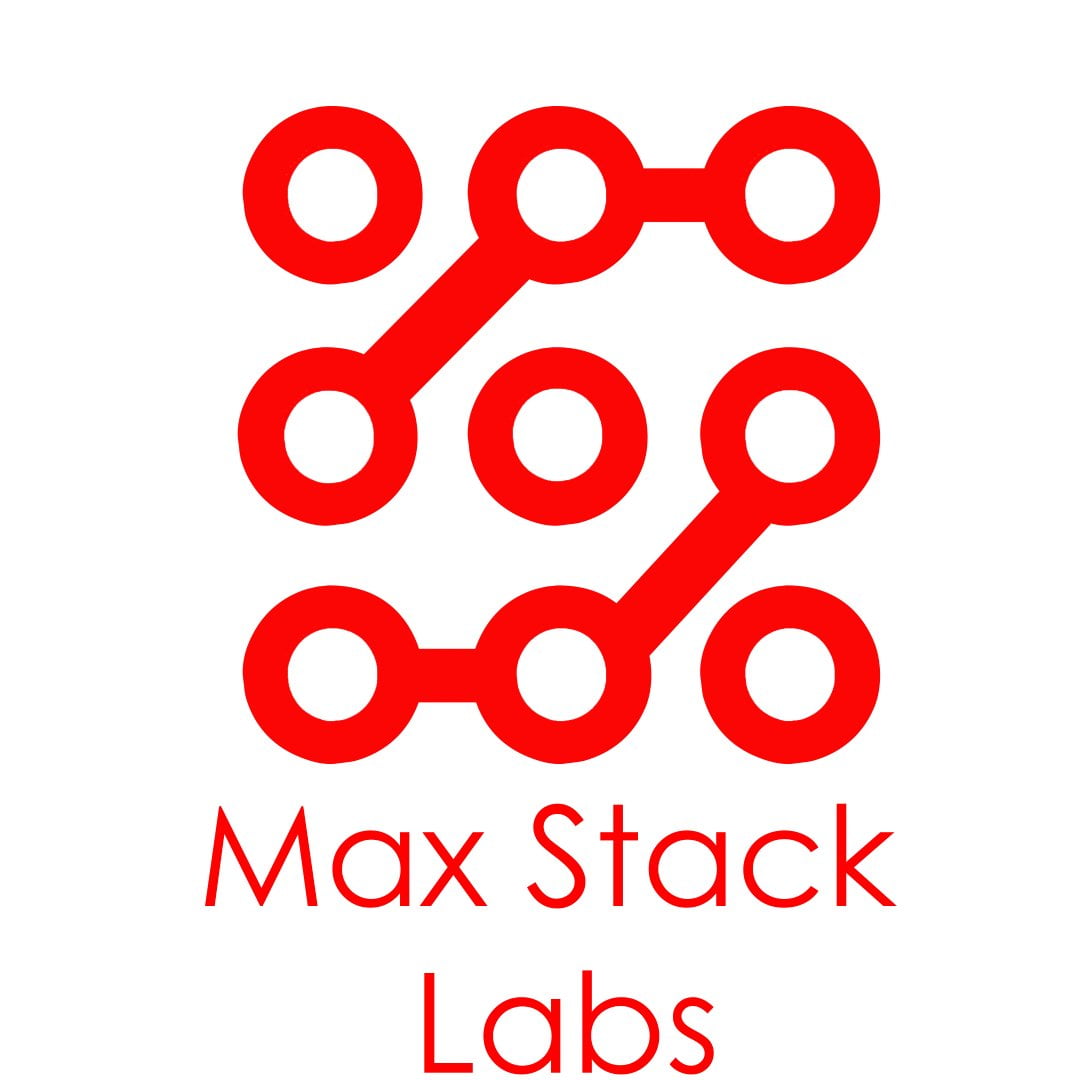
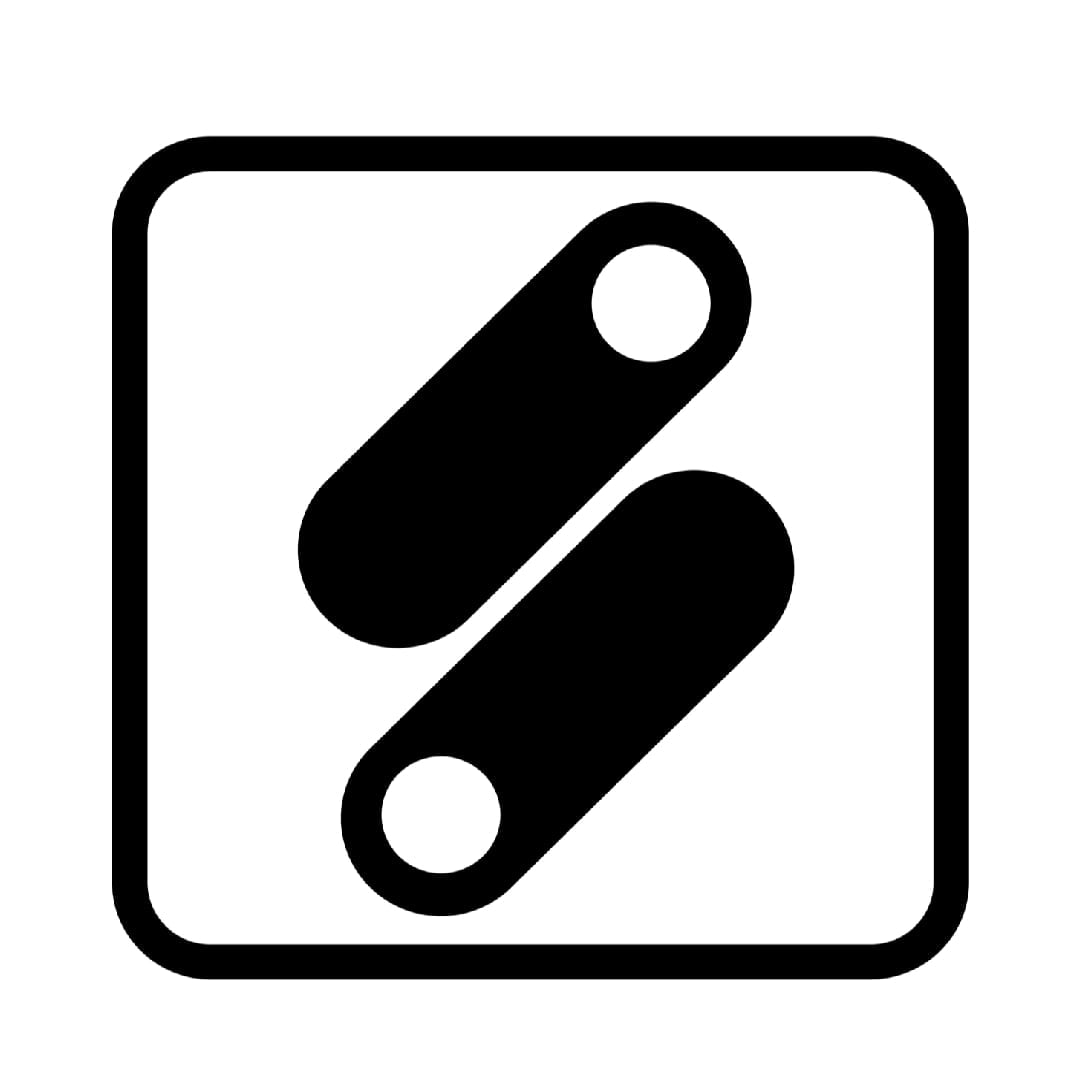
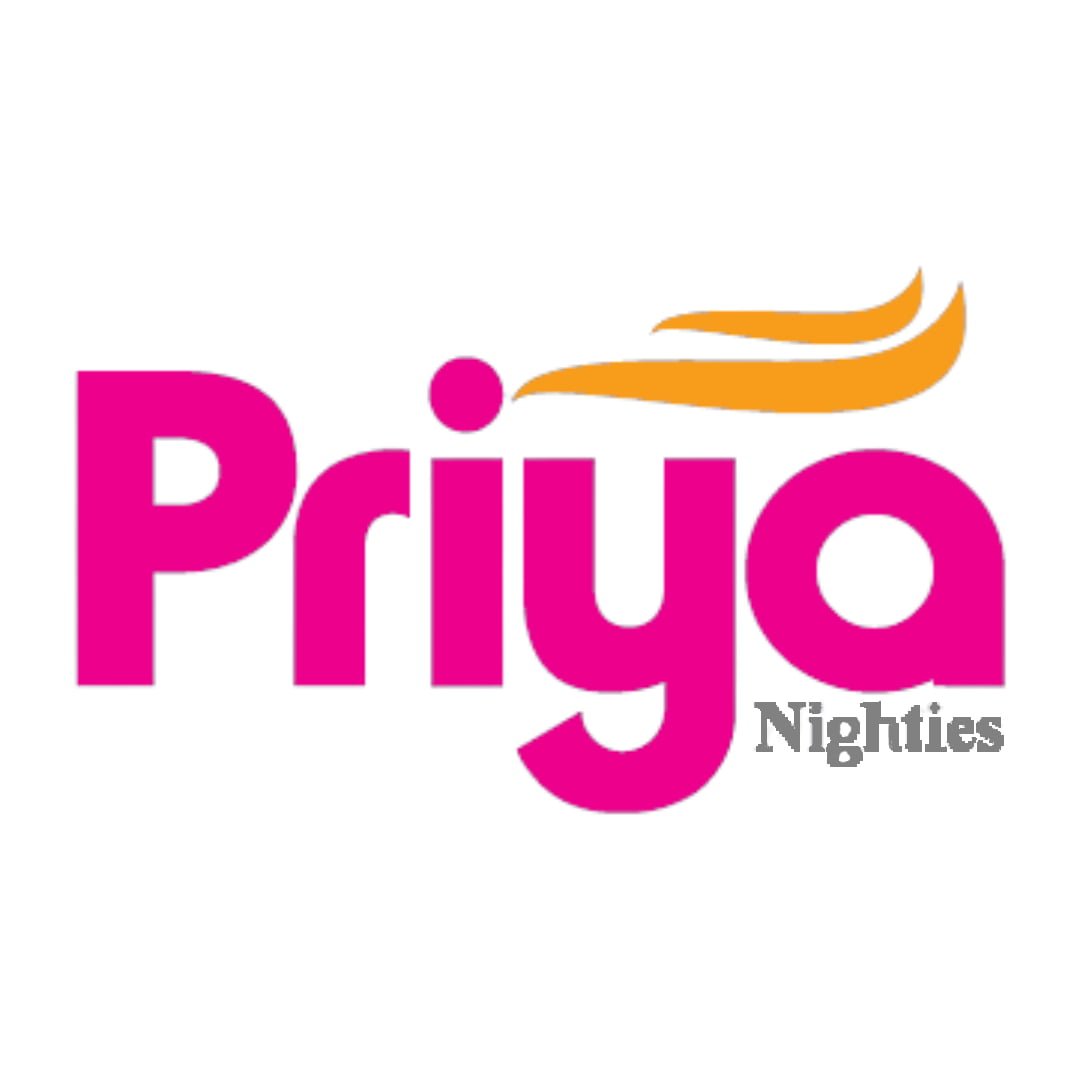
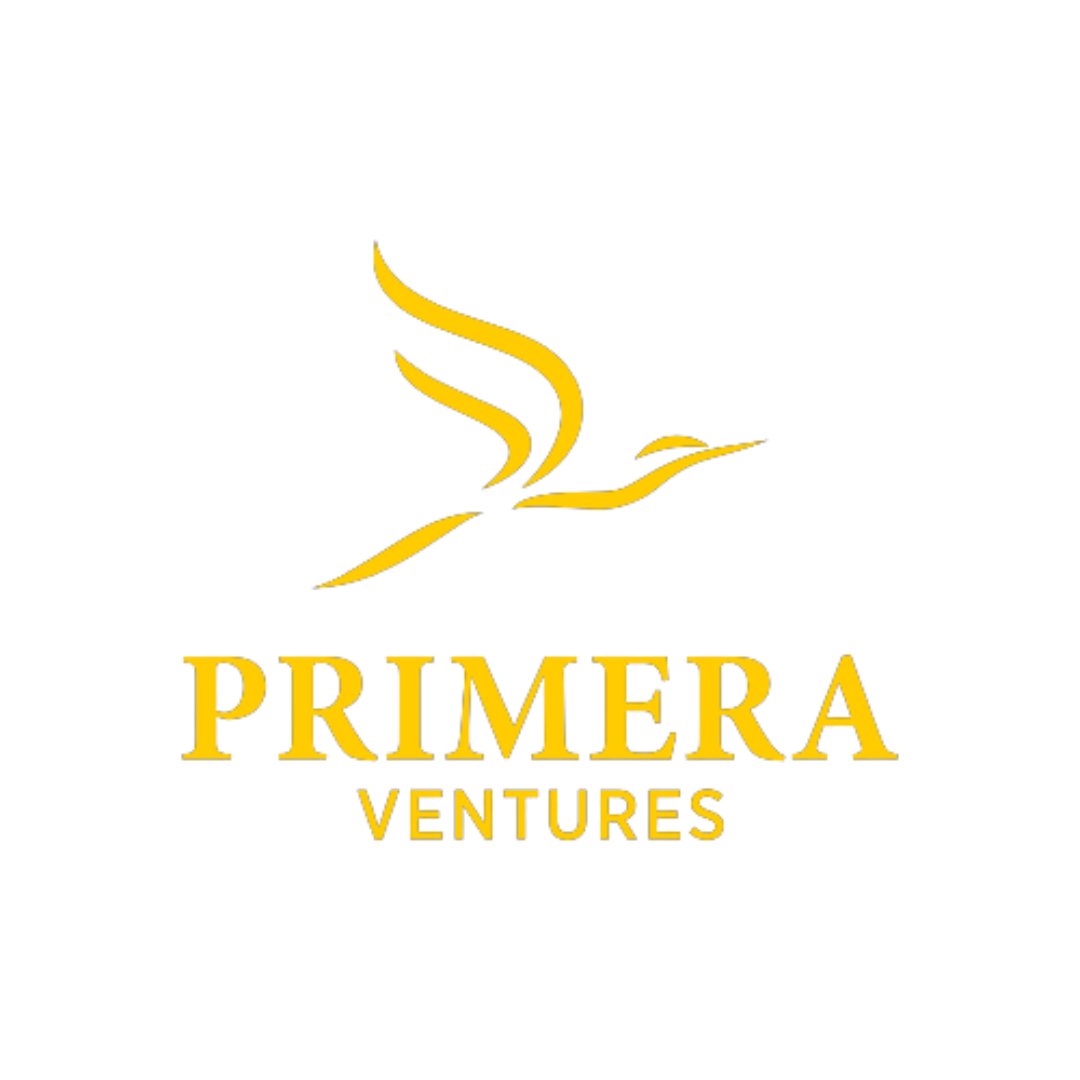
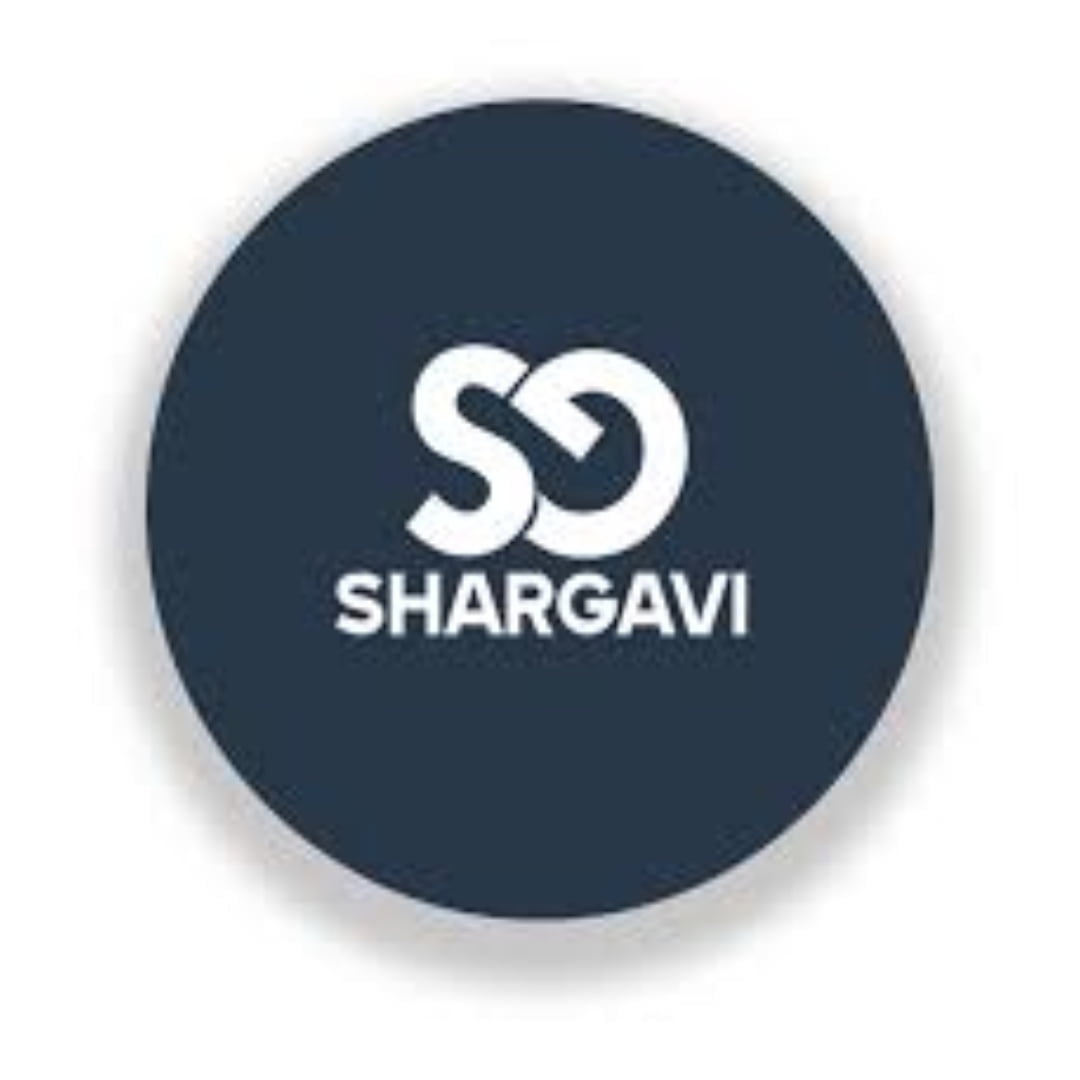
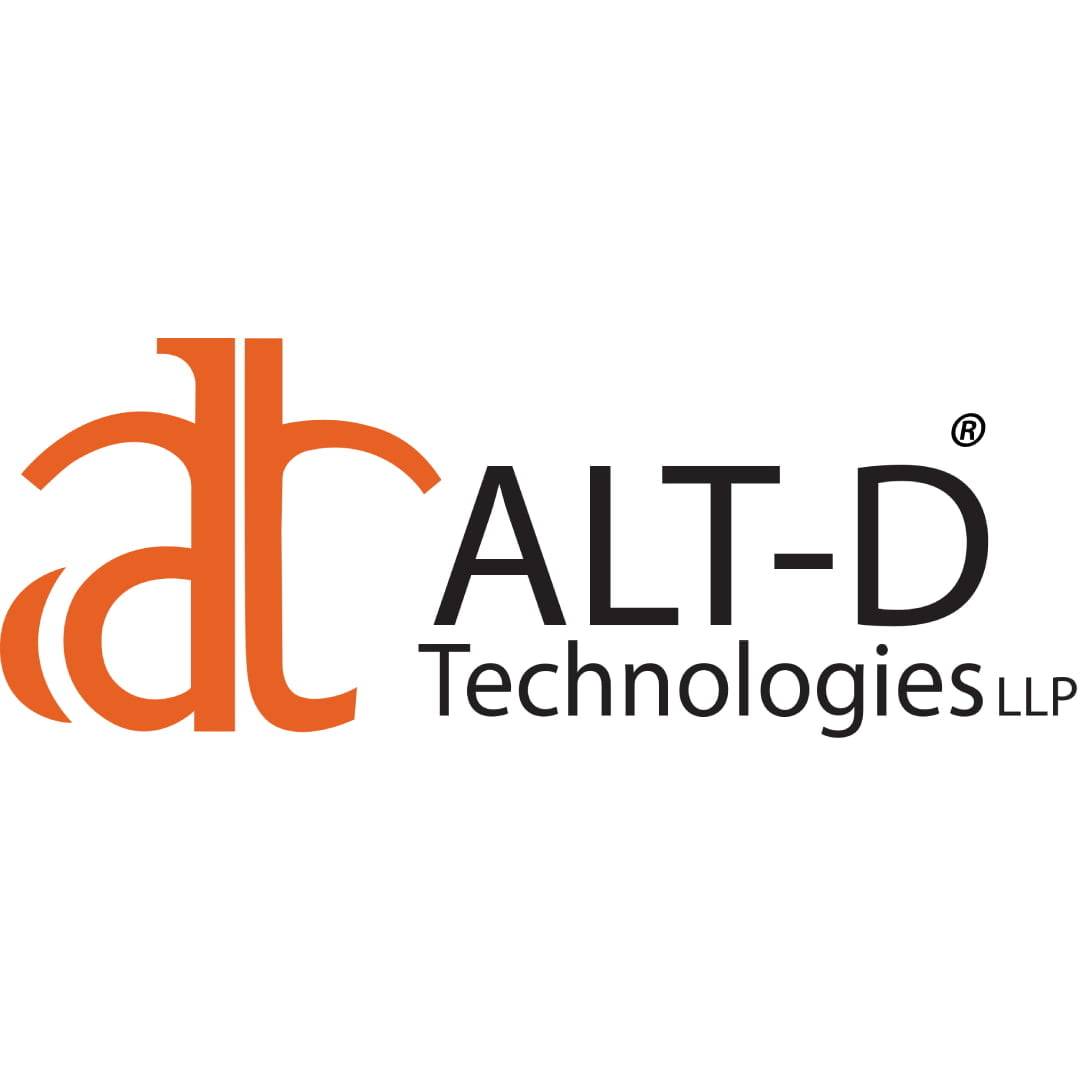
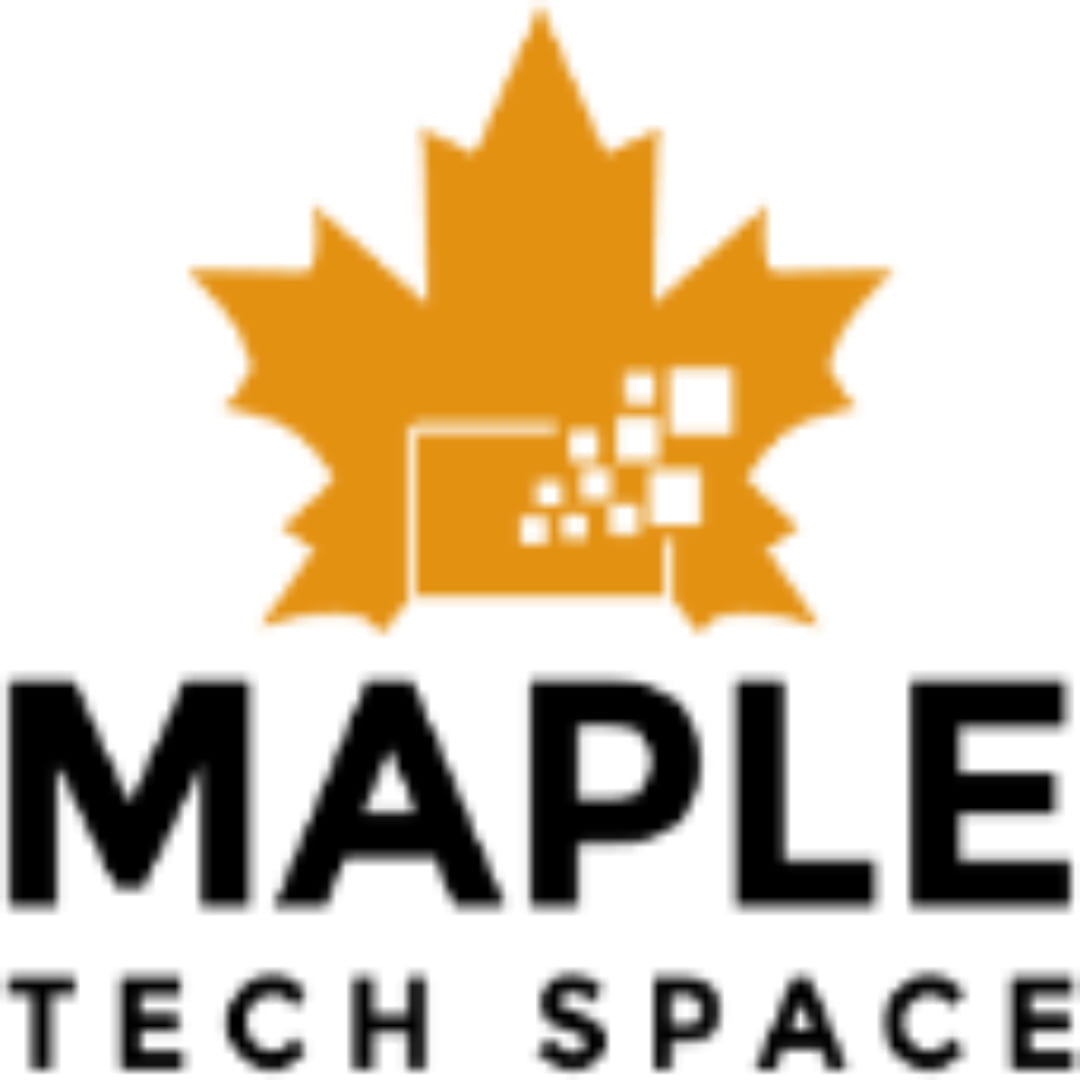
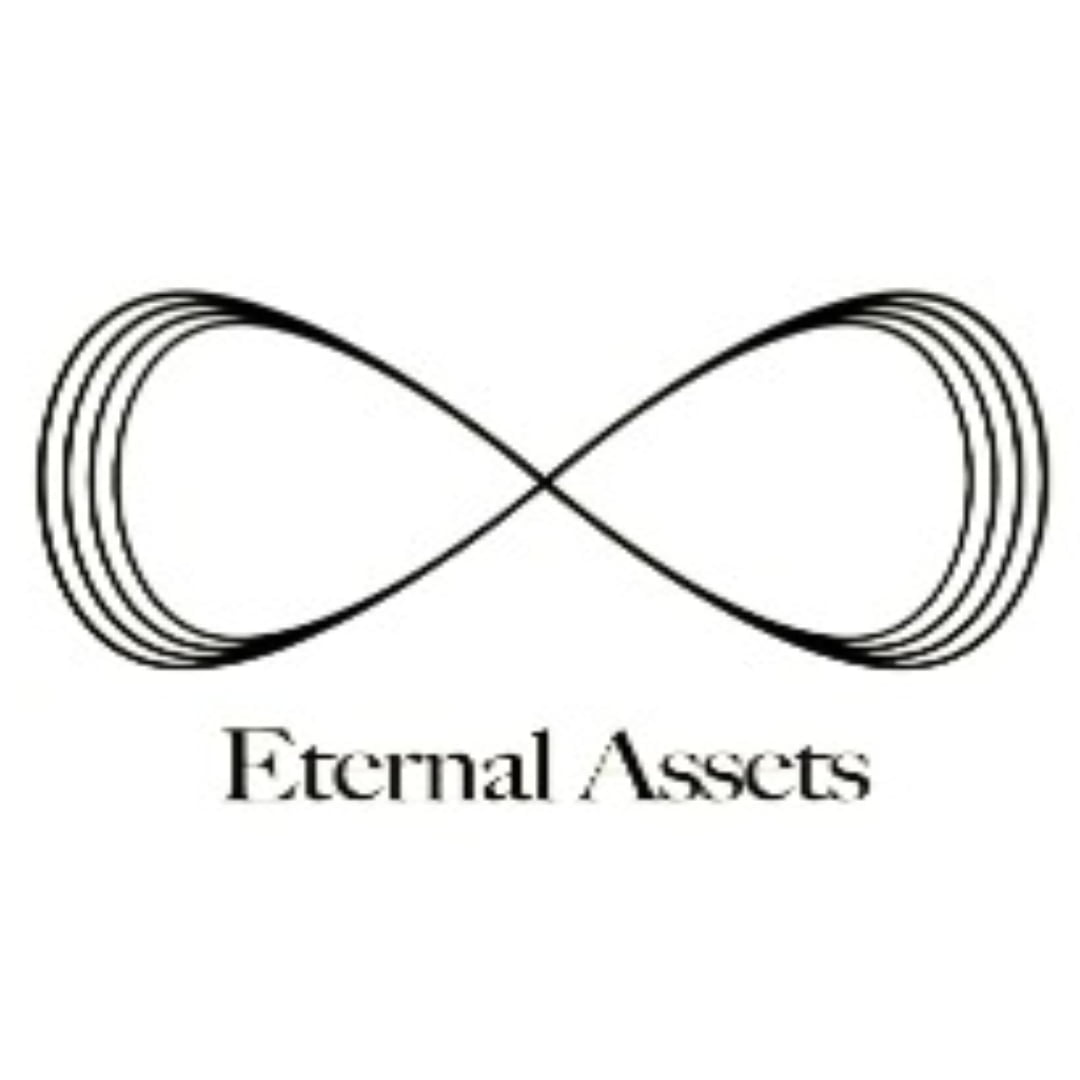
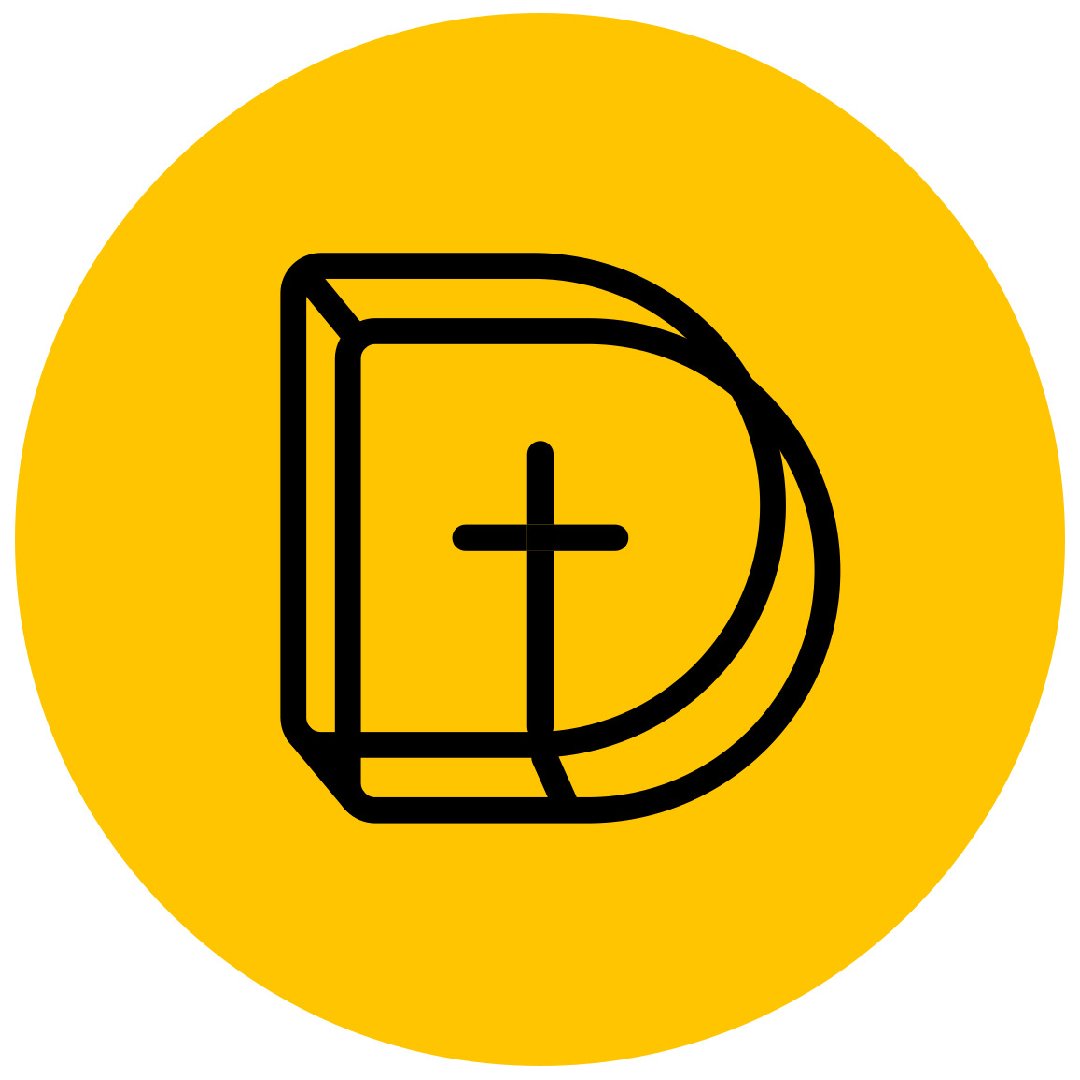
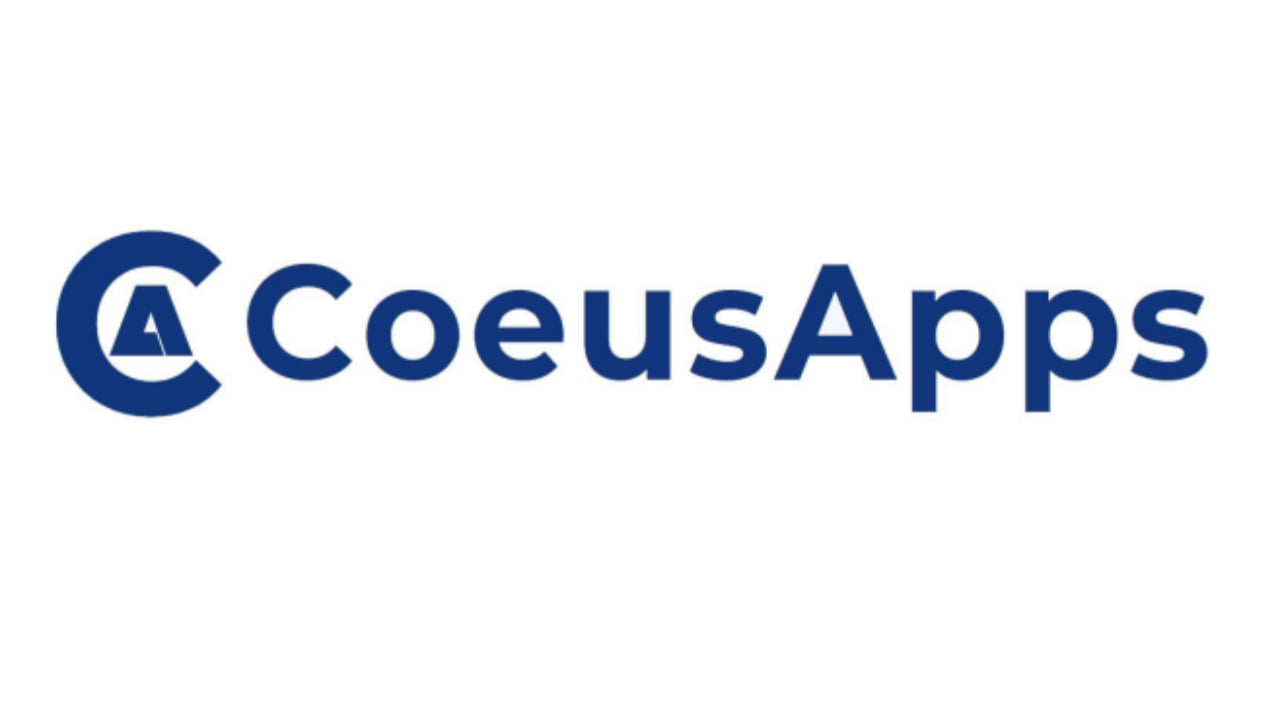
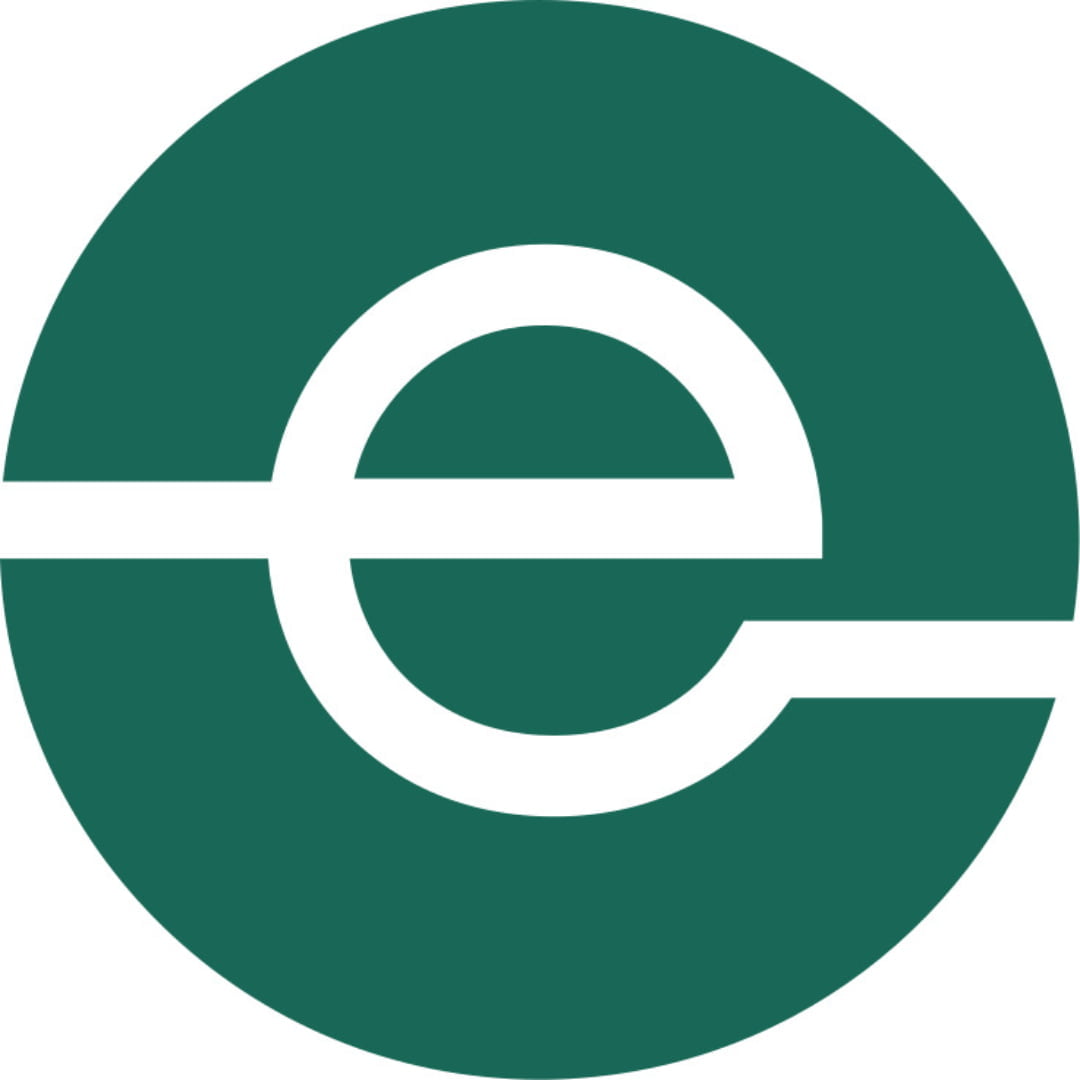
Connect Us